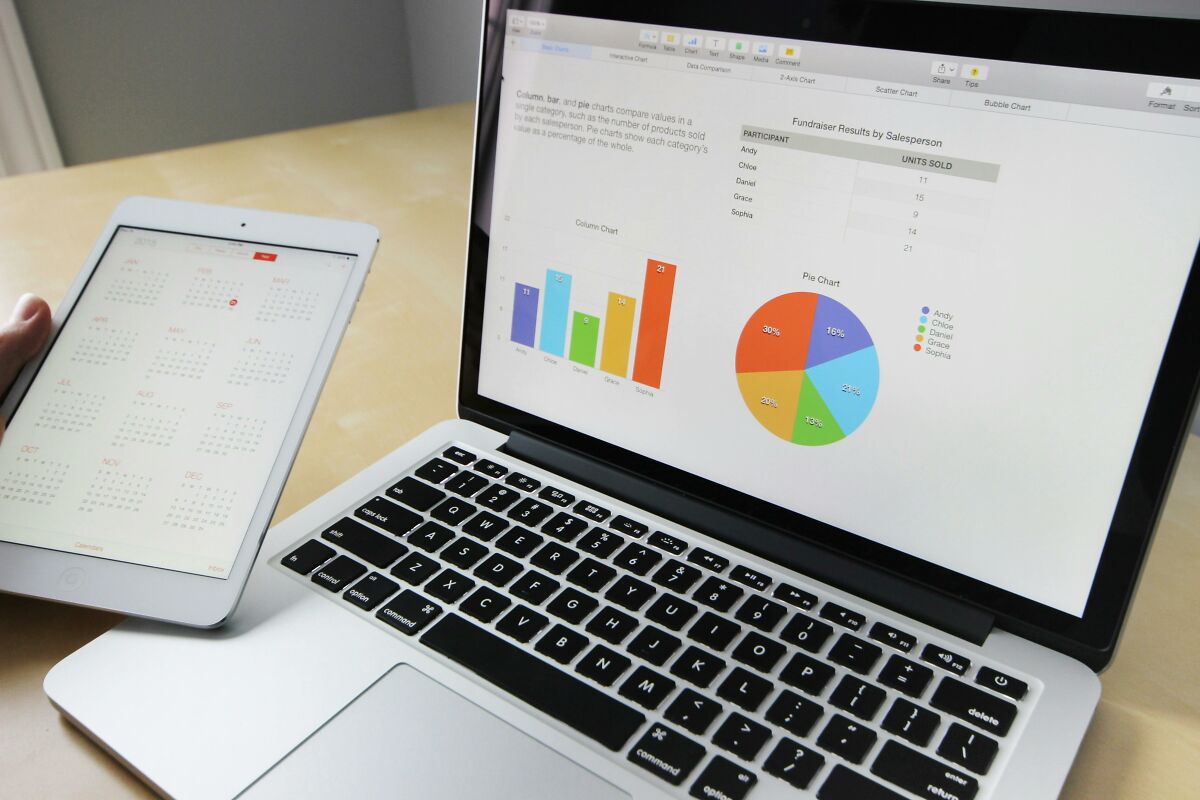
Exploring Clinical Trial Data For Effective Utilization In Future Research
Clinical trials test behavioral, medical, or surgical interventions in populations. These trials are researchers’ primary mode of determining whether a new method of prevention or treatment, like a medical device, diet, or drug, is effective and safe for use by people. Ordinarily, clinical trials assess a new intervention’s effectiveness or harmfulness relative to existing interventions.
Over the last few years, clinical trials have adopted technology at an all-time high. The increased use of technology and complexity of study designs have generated massive volumes of data. This data could help researchers accelerate trials, fast-track the development of effective treatments, and better serve patients. However, the sheer volumes are overwhelming, and concerted efforts must be taken to harness the data for future research.
More info: Clinical trial site management
Image credits: CDC
Present State of Clinical Trials
Technology continues to play a huge part in shaping future clinical trials. However, we must first understand how to better deploy it and manage the information it generates. It’s interesting that despite the heavy tech uptake in the sector, study timelines keep going up. That is likely because Clinical Research Organizations (CROs), sponsors, and research sites are overwhelmed by the volumes of data they must gather, clean, and scrutinize in clinical trials. For instance, a typical modern-day phase three trial involves approximately 3.6 million points of data. That is at least thrice the data they had to deal with a decade ago.
Besides the enormous amounts of data, studies have become more complex. Sponsors register numerous adaptive or multiphase studies, which plan for changes mid-trial. Also, the COVID-19 pandemic occasioned hasty adoption of technology to sustain the many new trials. Stakeholders implemented several eClinical tools with limited change management aid. That led to a widespread crop-up of inefficient technology.
Increased research complexity and data points coupled with rushed adoption of technology created a perfect breeding ground for the present challenges in clinical trials. To make the most of all the data we generate from trials, we have to review our strategy for adopting and deploying technology.
Employing Clinical Technology to Better Data Quality
The central technology employed in clinical trials involves active and passive data collection. These modes of gathering data yield better quality data because of their timeliness. Historically, patient data was never considered while making decisions until trials concluded. Due to present-day real-time electronic collection of data, quick adjustments can be made mid-study.
Passive Collection of Data
This data collection approach is also known as automatic data collection. It improves the quality of data by eliminating errors common with manual entries. Such errors could arise when a participant accidentally enters the wrong details or remembers something after submitting an entry. These mistakes affect the overall quality of data.
With passive collection, the subject does not need to key in the information. Technology such as wristbands, smart watches, sensors, and other devices automatically capture the required data. The real-time collection and relaying of this information makes this approach highly accurate. What we require is a well-synchronized system for managing this data to ensure it’s not scattered about.
Active Collection of Data
This approach requires a researcher to digitally enter the data collected from primary points. Unlike the passive method, active collection does not do away with errors from manual entry. Still, it may offer checks on data during entry, eliminating errors in transcription that may arise while transferring paper information to electronic systems.
A reduced likelihood of errors means better-quality data. To maximize the quality of information gathered from this approach, tech vendors and sponsors should collaborate with experts in health literacy to ensure patients understand their eClinic framework. If a study participant misinterprets a prompt and responds per their incorrect perception, you get errors and poor-quality information.
The types of active data collection in use today include electronic case report forms and electronic patient assessments/diaries.
Image credits: Pixabay
Better Utilizing Clinical Trial Data
The passive and active technology employed to improve clinical trial data does just that. However, as reiterated throughout this piece, we need particular strategies to realize this data’s potential. Here are some steps we can take to make better use of the massive data generated from clinical trials:
Employ Standardized practices of Data Collection Across Trials
Standardizing data collection practices across trials is critical to the accuracy, reliability, and consistency of data collected. One way of standardizing data collection is using the same terms or naming conventions. This includes measurement units, terminology, data types, and date formats. We should also use standardized codes for classifying or categorizing the trial data. This allows for easy analysis and integration.
Also, collaboration and communication must be clear and deliberate among all stakeholders in the sector. The lists of vocabulary, units, and other standard parameters should be shared widely to make the cleaning and scrutiny of the enormous amounts of data easy. Lastly, all information gathered should be converted into a standard electronic format for more efficient analysis of data from disparate avenues.
Compare Portfolios Across Studies
Standardized collection of data also facilitates robust analysis tools, making it possible to have big cross-study data lakes. These vast databases can aid sponsors in cross-evaluating their entire portfolio, producing evidence for decision-making across several trials.
Develop Platform Technologies
Developing sturdy platform technologies is also an excellent strategy to manage clinical trial data better. Clinical trial site management platforms, whether developed in-house or by third parties, have been pivotal to improving the usefulness of trial data. Instead of coming up with a new ecosystem for clinical data for every trial, we can deploy a standardized platform technology through several molecules of a specific therapeutic area or disease. That will slash study durations and improve research efficiency.
Embrace Enterprise-Wide Adoption of Technology
Lastly, we must ensure the technology deployed for clinical trials is adopted enterprise-wide. Otherwise, all those massive data set we collect won’t be valuable. Technology is undoubtedly the present and future of clinical trials. If we adopt technology for some trials and fail to do so for others, we do not achieve the standardization we desire to streamline our data streams and ease analysis.
However, this adoption should not be rushed. It should begin with a re-examination of our tech needs, identification of gaps, review of existing tech, and thorough staff education. Once these are clear, scaling and future-proofing are essential to successfully harnessing data for efficient use in future research.
Permanently Harnessing Clinical Trial Data
Quality data always has the potential to improve future studies. Subsequent trials in similar fields often benefit from previous ones. The more data we have on a disease or intervention, the more likely we are to know what works and what should be dropped to improve patient outcomes. Unfortunately, at the moment, most data streams for clinical trials are not adequately synchronized. Although we have tons of valuable and quality data, we cannot fully exploit it until we effectively leverage technology to help us manage it better. To attain this, we must standardize our data and enhance its reproducibility.
Final Thoughts
The harmony between data science and clinical trials ushers a new era in healthcare research. By taking advantage of AI, advanced analytics, and big data, we exponentially expand our potential to understand complex diseases and discover new interventions.
385views
Share on Facebook
14
0